AI Metrics That Matter
with Jon Tucker, CEO of HelpFlow
In this episode of Ecommerce AI Mastermind by HelpFlow, Jon dives into how AI can prepare businesses for the intense demand of Black Friday and Cyber Monday (BFCM).
Topics include AI-driven forecasting for customer service volume, automating tickets, and stress-testing AI systems for peak performance. Jon also discusses how AI can identify sales opportunities and optimize cross-selling.
The episode wraps up by emphasizing AI’s role in providing seamless customer service during the high-sales season.
AI Metrics That Matter
with Jon Tucker, CEO of HelpFlow
Your customer service team is your company’s first line of defense. In order to have a lasting, successful business, it is paramount that your customers are treated with the utmost care and respect. When you provide quality customer service, you create a positive environment for your employees, who then, in turn, take their positive attitude to interactions with customers. Furthermore, happy employees make for happy customers! Join this conversation with Michael Potters as we dig deeper into that. We will discuss how to make a customer experience awesome while also leveraging it to be a big revenue driver in your business.
Michael is the Enterprise Partner Manager at Gorgias, a multichannel helpdesk for e-commerce brands. Gorgias empowers over 7,000 businesses with better customer service on platforms like Shopify and Magento. Before Gorgias, Michael was the Co-founder and CEO of Parachute Coffee, a coffee subscription service that LeJeune Brands acquired in 2020. Outside of work, Michael volunteers at King’s University College as an Alumni Association Board Member, leading the outreach and coordination for the Toronto Alumni Chapter.
Learn more by joining us at the DTCX San Diego on September 28, 2022 to hear from the founders of brands and tech apps. This is an in person event where you will have time to network with brand founders, and learn the current industry trends.
The event will take place at Wine & Culinary Event Center, San Diege, CA, starting with panel between the TOP Ecommerce experts from Gorgias, Helpflow, PostPilot and Sendlane.
In This Episode, we discussed:
- [00:01] Introduction to the show
- [02:21] Today’s Topic: Customer service
- [02:54] Quick bio of today’s guest; Michael Potters
- [04:14] A bit of Michael’s journey as a merchant
- [08:02] What’s special about Gorgias that’s unavailable in other customer service providers?
- [11:40] How Gorgias handles its operations to produce a 30% success rate in eCommerce stores
- [18:09] Tactical ways that eCommerce brands are using to maximize their customer experience
- [23:26] Additional third-party tools integrated into Georgia to ensure its efficiency
- [30:10] Golden key takeaway nuggets from Michael on
- [32:40] You can start a free trial with Gorgias today and see how it works
- [36:02] Wrap up and calls to action
- “Gorgias’ customer support agents are 30% more efficient than other providers.”
- “If you are able to solve your customer concerns within six hours, the estimated revenue impact is about 1%.”
Hey guys, welcome to the Mastermind. We're just going to do a quick sound check, make sure everything is stable here as we get started, and then we will get going. Racel, if you can let me know in Slack over here that audio video is everything good like we had right before, and then we will go ahead and get started. We're super, super excited to dig in.
I know we've got a lot of different people on different channels, whether it's social media or directly in the directly in the webinar room, but we're going to be covering a lot of stuff today. The reason why we do these webinars is to make it so that you have tactical ways that you can apply AI in your own business. And today we're going to be talking about a customer service specific situation. That is the business we are in here at Helpful, as many of you know.
But in order to apply AI in your business, you really need like two separate things is the way I would think about it. Number one, you need really specific tactical guidance, like how to specifically do a certain thing. And so today, like you can see on the screen, we're gonna be talking about how to measure AI success and customer service. So like when you're doing customer service and you're using AI, like how do you measure it?
We're gonna talk very, very tactically about how to do that. But stepping a little bit back in order to really get really strong results in your business when it comes to AI, you need to be AI minded is kind of what we call it at Help Flow. And what AI minded means is having an understanding of what's possible with AI, having at least the high level understanding of how different AI technologies work, Because what that will start to do is you will identify and kind of think of ways you could apply AI. So when you're AI minded in your business, you will start to come up with ideas of like, oh, maybe like chat GPT could do that.
Or maybe, you know, an open AI assistant could do that. Or maybe some AI tool is out there that can do this thing or solve this problem in your business. When you're AI minded, you will be able to identify ways to apply AI in different use cases, in different situations. And so that is the goal of what we want to do.
We want you to be able to be tactical in applying AI in your business, but we also want you to be able to think of additional ways to apply AI. As we get started, just so we understand kind of the makeup of everybody in the audience, if you can comment in the actual comments, regardless of where you're at. So a lot of the comments will come into the webinar system that we have here in front of me. But comment in of where you're coming in from.
We're in San Diego. We've got team members all around the world. But just comment basically on where you're from, whether it's LA, New York, France, all over the place. um it's always interesting to see where people are at um if you can drop that in the comments um and then second input um on a scale of one to ten how deep in the ai space are you so on a scale of one to ten how deep in the ai space are you how experienced with ai are you you know one being like I have no idea I've heard chat gbt but that's it I've never even used it right uh and maybe a ten being like you're building stuff that actually produces value in your business so roughly where are you on that spectrum one no experience at all ten I'm building stuff that's providing value like I'm here to go you know levels and levels deeper If you can comment in the comments with that, that'd be really helpful to basically see just an understanding of where everybody's at.
Before we dive into the topic of the day, one of the things I want to do is share some crazy stuff that we're seeing with AI in the space. That's one of the things we want to do with these webinars is help you understand what's possible with AI. What is possible when it comes to AI so that you can start to say, oh, I think this might be doable with AI, right? This problem in my business might be able to be solved.
So I always try to share like one or two crazy things we're seeing in AI that's now possible. And then second, I try to share just kind of general news stuff just so you can have kind of a pulse of what's going on. So two things that are pretty crazy that are happening in AI right now that I think will be new to many of you, depending on how deep you guys are in the space. But I want to be able to walk you through this.
So one is a concept called agentic AI, agentic AI, which basically means individual AIs working together in collaboration to accomplish a goal. So individual AIs work together in collaboration to accomplish some sort of like complex goal, right? and I don't have slides for this particular part I typically don't for like the news and those types of things but I want to walk you through what I mean by this so take chat gpt as an example like I assume most of you have have been in chat gpt for something right if you give chat gpt a really broad goal that's like super complex right so close the sale with this prospect here's the prospect here's our service close the sale like get them all the way to the point of like closing the sale right that would be really really hard for chat gpt to accomplish because it's such a broad request, there's so many details that will influence the strategy to be used.
And you're just giving it one hit and saying, go close the sale, right? It's very broad. But if you break that into individual pieces and then ask ChatGPT to do each individual piece, it's much more attainable. And when I say ChatGPT, I'm using this as an example, like interchange ChatGPT with AI, like technically you wouldn't use ChatGPT for what I'm about to say, but it'll help you visualize it.
So if you break a complex goal into individual steps, then ChatGPT will perform a lot better. And so what agentic AI enables you to do is basically have AIs that work together on individual narrow pieces, but they're all coordinated and aware of what each other is doing, what the other AIs are doing to accomplish that ultimate goal. And they are managed by an AI. that knows what each individual AI is doing, and it keyways those steps and kind of sends it back to an AI and says, hey, you need some more information, for example, right?
It can even escalate up to a human for feedback. So it can say, hey, I think this is the best way to prepare for the sales call. Can you give me some feedback on these two points, right? Or can you give me some general feedback?
So it could be objective like, A, B, or C type of feedback, choose one of these options. Or it could be subjective of like the human, you know, giving some coaching back to the AI. The key thing I want you to take away from this is that you can have AIs working together in collaboration, managed by an AI to produce a complex output. That is essentially a department.
in a company. That is essentially a team. That is exactly what humans do together is there's individual specialists that work together in collaboration, sometimes managed by a manager, and they do complex stuff, right? So agentic AI is something that's starting to become a lot more... attainable for companies to build with.
A lot of the tools that you're going to see out in the marketplace, including the customer service tools, they are built in frameworks that are agentic AI frameworks, and they are extremely powerful. So I just wanted to share that as an example of kind of what's starting to happen in the AI space. Very, very powerful for building tools. The second thing I want to mention is AI-assisted coding tools.
There's a lot of tools coming out that give you the ability to create complete software products or complete software tools very quickly, even if you don't know how to code. I'll rush through this example because we could get pretty technical on this particular one. But ChatGPT, for example, has been pretty good at creating code for simple situations. For example, narrow, you know, spreadsheet macros, you know, narrow Python code.
If you know Python, like it can do very narrow coding stuff very, very well. And it can do relatively complex coding stuff pretty well. But recently, something came out from Anthropic, which is like a competitor to OpenAI, which is essentially a new model. It's called Claude, a three point five sonnet.
It's basically a really, really good AI model that is great at coding. Very, very good at coding to create pretty complex software software code and. What has changed in the AI space is you have Claude that's come out that's very good at coding. And then you have a tool like Cursor.
There's a couple of these tools out there, but Cursor is a coding tool that is basically designed to integrate AI and have AI create your actual software code for you. And so basically what Cursor enables you to do is give AI the context of all your code and then basically integrate AI directly into the coding process so you can highlight and modify code You can highlight and add to code. You can have a chat interface on the side that's in cursor, has access to all your code and say, I want to create a tool that does this. It will literally tell you, first you need to create the file, then you need to add this code, then you need to do that.
In the interface, it's designed to actually execute those things. It'll tell you, you need to create these files and do this code. Then you literally press these play buttons and it will create the files and create the code and do everything. Remember, it has context to all the code.
So I bring this up because I think it's something, especially for leadership teams that are on the mastermind or watching this after, this is opening up a lot of development capabilities within Teams for Teams that haven't historically done a lot of custom development. In the past, you've had to hire developers to do everything. You need to know how to manage developers. It's pretty complex.
It's doable, but it's complex. Now we're at a point where you can start to at least modify existing code fairly easily. You can also have team members on your team that have technical mindsets, but maybe aren't coders, start to actually build stuff. And we've done that at Help Flow.
We've done that in other lines of business and it's working extremely well. And myself, I'm fairly technical. I know how to architect code. I know how at a high level to read code, but I cannot write code from scratch.
I've been able to build agentic tools and prototypes fairly easily using Cursor and it's extremely powerful. Definitely something to consider digging into is AI-assisted coding. Building software is becoming a lot easier, not super easy, but a lot easier than it used to be and it's because of AI. Those are some two crazy things that we've been seeing.
There's a number of other things happening in the AI space, but those are the main ones I wanted to share today. um just so you can kind of get your mind thinking of like oh my gosh like what might be possible if you could have a bunch of ais working together in an agentic system that'd be pretty valuable what might be possible if you didn't need to hire an army of software developers to build this idea that's in your mind you just needed to hire somebody that knows how to use cursor or learn yourself right you don't need to learn every aspect of coding you need to learn how to code with ai So a lot of different opportunities happening in the market.
But today, I want to give you really tactical guidance on a specific topic, which is how to measure AI when you're using customer service. When you're doing customer service, how do you measure if the AI is doing well, if AI is managing the customer service process. And a lot of people struggle with this. Monitoring the effectiveness of AI and customer service, it can be complex if you don't understand the metrics and how it works.
And the reason for that is, number one, AI is really new. It's tough to know how it works. It's complex. When people say AI, they kind of mean different things at different times.
And so it can be fairly complex just because it's new. Second, there's a ton of different tools. There's a lot of different customer service tools for AI, and they all track the metrics slightly differently. You want to make sure that you are aware of which metrics to track and the foundation of how to track customer service success, regardless of what AI tool you're using.
There's a lot of tools that can make it complex. Then lastly, if you don't manage the AI really well, it can really negatively impact customer experience. People are already frustrated at times by talking to bots and having these continuous press one for sales, press two for support, those types of things. And so if you make it feel like a robotic experience, it's gonna perform really, really bad.
You're better off having like an email form rather than a poorly executed bot. With that said, if you know how to track AI and you know which metrics to track, it can be as easy as tracking a human CS team. And so that's really what I want you to get out of this today is knowing how to monitor the effectiveness of AI in a customer service process. And that is part of what we do at HealthFlow.
So we have run customer service operations for hundreds of e-commerce brands. We've done it for over a decade. And so we've been in this game for a long time. We've seen a lot of different technology innovations and evolution.
And in the last three years, specifically, we've gone very deep into AI, not only in the customer service space for our services and what we do, but we have a lot of AI stuff of what we do on backend operations. We even build our own tools. I mentioned the cursor tool. Part of the reason why we got way into that is our team literally builds tools.
And so we're very technical. And by...
Going deep into AI over the past couple of years and having this environment of many different types of clients, many different types of tech stacks, we're able to really understand how to best apply AI in different situations. And that really sets us apart from other agencies that are kind of reactive to AI. Like everybody in customer service is doing something with AI because they have to. We've been doing it for, you know, call it around three years because we're just way interested in the tech.
We've always been tech forwarded company. And so it's been a really exciting time. But today, what I want to walk you through is the mix of metrics we use to track the effectiveness of AI and customer service so that you can really use it effectively in your business. If you were on the last webinar, you might remember this concept.
This is something I frequently go back to with business leadership teams is. Before thinking about which metrics to use and how do you track them, I want you to just shift your thinking when it comes to AI in general. Think of AI as a human. And in this particular case, think of an AI customer service system as a human customer service agent.
Basically, what's going to be happening is the AI is going to be processing tickets. You need to measure the accuracy of the answer just like you would with a human, right? So you need to QA it. You need to look at the customer satisfaction.
You can do this with your normal QA process. You can score AI the same way as you do with your human team. And then you can add on some AI specific metrics to really optimize performance. But the key thing I want you to just be focused on, not only in today's session, but really like any conversation about AI, don't think of it like software.
Like think of it like either a human, an individual human, right? Or... like the agentic thing I mentioned at the beginning, think of it like a team of humans, right? But think of AI as a human because you manage it and train it and optimize it in a very similar way as humans. So first, the first thing to track when it comes to doing AI with customer service or doing customer service with AI is CSAP.
You need to measure the customer satisfaction score of tickets that are done by AI. And so a high customer service satisfaction score basically means that they had a good customer experience, right? It's kind of like in the name. And so what you need to look for is having the scores be consistent with the AI tools When you compare them to your human customer service tickets.
So if the human agent does a customer service ticket and the typical score is, you know, eight point five, which I'll get to in a second. the AI should be at least eight point five or above, right? And so the best practice, it depends on how you measure it. Everybody measures CSAT a little bit differently, but in general, it should be eighty percent or higher.
We like to see it ninety percent or higher, but basically eighty percent or higher is what you should be seeing. But the key thing I want you to understand is if you over automate with AI, if you're doing too much with AI, what you're going to see is lower CSAT scores on the AI tickets specifically. and declining overall CSAT in the entire business. So CSAT ultimately measures how happy are people with the way the ticket was handled, whether it's a human or with an AI ticket.
And you want to make sure that the CSAT stays as good or better when you implement AI. So in terms of high-level how to measure CSAT, I'll brush through this fairly quickly because I think most of us will be familiar with this. We can definitely hit it during the questions as well. And by the way, if you have questions as we're going, go ahead and put it in the comments and I should be able to see those come through.
If you're on a different platform where we're streaming this, I'll have my team put it in Slack, Raycel and Angel. If you guys put the questions in Slack, that'd be good. But in terms of how to measure customer satisfaction score, it's pretty straightforward. Number one, you're going to collect feedback from customers after the actual customer service interaction is done, right?
So you can use different survey tools. A lot of help desks have this built in directly, but basically you want to collect feedback from them. And typically one of those feedback items is a rating, typically between one to ten of how satisfied are they with the outcome? You can ask this question a couple of ways.
You could technically ask, how satisfied are you with the speed of response? How satisfied are you with the solution provided? You can break it up a lot, but basically what you're doing is you are calculating the average CSAT score and then basically seeing how that score differs between human customer service agents and AI customer service agents. So again, you're measuring CSAT already, or you should be in your customer service team.
You do the same exact thing with AI. You measure the CSAT on the AI specific tickets. The second metric is automation rate. And so this is where we start to get into the AI specific metrics.
But this is very similar to the metrics you would use for a customer service agent. If you had a customer service agent and they couldn't handle any tickets on their own, they handle zero percent of tickets completely on their own. then you would measure it in a similar way. And so that's essentially what we're doing with the automation rate.
I'll kind of zoom out because I think that's a little too esoteric. But basically what automation rate is, is the percentage of tickets handled by the AI automatically without escalating to a human. And so basically, if you're able to have a high automation rate while keeping the CSAT stable, right, so the customer satisfaction is good, but the automation rate is high, then you've got a well-executed AI strategy. What we often see with clients is when they come to us, they've said, you know, we've tried AI and like it's not able to handle a lot of tickets, which I'll get to in a second.
Or they say, we're crushing it with AI. We've got, you know, seventy percent of our tickets handled by AI. But then when you look at the CSAT scores, they're abysmal for AI specific tickets and even for the whole brand. Or they say like, oh, yeah, we don't measure CSAT.
Like, I think it's pretty good. And so if you if you don't measure the CSAT and you have a high automation rate like that, that's a recipe for disaster because you can automate a lot of tickets with AI. But if the CSAT is bad, it's going to crush the business. what you want to do is you want to target an automation rate of fifty percent or higher um and that's like the target that's not the the the initial threshold you're going to be able to get to typically you're going to get to thirty percent relatively quickly then get to forty and fifty percent as the next milestone you can get it much higher than that but fifty percent or higher is kind of like the measure of success uh for an automation rate and then you want to measure the escalation rate separately which I'm going to get to the escalation rate is the percentage of tickets that an AI starts that then ultimately has to go to a human.
We'll get to that separately in a second. But automation rate is the metric to measure the percentage of tickets that the AI is actually handling. The way that you measure automation rate, you're gonna track the total number of interactions handled by the AI tool, and then basically figure out which ones, the number of those that are resolved without escalating to a human, and that turns into your automation rate. And so what you're trying to do here, again, is to say how productive is the AI?
Like what percentage of our tickets is AI handling on its own? That's the automation rate. The last one, and then we're going to do a bit of a recap, is escalation rate. And so escalation rate is essentially the percentage of tickets that the AI started and then had to transition to a human, right?
So it started the ticket, but it wasn't able to finish it, and it went to the human. Some escalations are expected for complex issues or predefined situations you want a human to handle. So maybe you have a pretty straightforward refund policy, but For VIP customers that have ordered more than five times in the past or over a thousand dollars of product or some number, you don't want to automate that. You want to have it just be handled by a human so that we can say, yeah, just refund it, right?
Or whatever the outcome is. It could be completely off process, but because it's a VIP customer, you want to just think very strategically about that and handle it, right? So some escalations are going to happen because it's complex, but some are going to happen because it's strategic to say, we just want to handle these with a human each and every time. The escalation rate, you should try to keep below twenty percent because that shows you that you're having AI handle as many tickets as possible within those CSAT boundaries.
If you start to have the escalation rate uh really really low and the automation rate really really high then um typically you're going to see csat start to go down you need to monitor that csat really really carefully um but for escalation rates specifically about twenty percent um keeping it below twenty percent is a good uh target um and I'll explain how to optimize these metrics in a second I know I've talked about how to measure them but I'm gonna talk about like how do you optimize uh escalation I'll talk about that in a second In terms of tracking, pretty straightforward. You're going to track the total number of interactions handled by the AI tool, and then you're going to count the number that were escalated to human agents, and that turns into your escalation rate.
So it's a function of the automated tickets compared to the ones that are escalated to a human. In terms of optimizing, let me touch on how to optimize each of these quickly and then we'll start going through questions. I think it'd be easiest actually for me if you can feed all of the questions into the Slack thread, that way I can just basically run through them there. That would be easiest because I know they're coming in from different channels, so start putting those in Slack now.
But how do you optimize the AI performance? Let's walk through some of that. First of all, CSAT, how do you optimize CSAT? This is done whether it's with AI or whether it's with a normal customer service agent.
You optimize CSAT by making sure you have like customer centric policies that are explained effectively and they feel like you're partnering with a customer, right? And so you wanna make sure they're fair policies and that you're communicating them effectively and in a nice way. You also wanna make sure that the responses are fast and the responses are thorough. So optimizing CSAT for AI is very similar to optimizing CSAT for human agents.
You're basically trying to make sure the customer is going to be happy with the response, right? Second, though, is automation rate. So in order to increase the percentage of tickets that AI can handle completely on its own, what you want to do is have a really effective or really robust knowledge base for the AI. So you need to have a strong list of situations and how to handle them.
You need to have that knowledge base crafted in a way where AI can can easily understand it, which is different than how a human understands. What we find with AI is you can be a lot more succinct and specific. The specificity matters more than all of the context, right? For a human, they often need like all this context of how to decide a certain situation or why it matters or the history of it or whatever.
With AI, a lot of times that's already in the model of like, why might somebody optimize for this outcome? And with the AI, you specifically just need to tell it the outcome. There's a happy median in between. It's a little hard to explain on a webinar like this.
But bottom line, you need to make sure that you have a robust knowledge base for the AI. And this is the key. You want to test the the knowledge base with variations of ways to ask the same question and then measure responses. So refund is an example.
I want to get a refund on my product. How does it answer the question? That's pretty straightforward. I'm not really happy with my product and I'm curious what you guys can do about it.
That's sort of the same way to ask, can I get a refund, right? Maybe they don't think they're going to get a refund. Maybe they think you're going to get a replacement or whatever it is, but you're asking the question, right? Or You're asking the question in a different way.
Or you can ask it in a really long drawn out narrative of how unhappy they are and why it happened and the person put it on the wrong patio and then it melted in the sun and blah, blah, blah, blah, blah, all these things, right? And the outcome is like, I'm asking for a refund, right? So what I'm telling you is have a robust knowledge base, but then ask the same type of question in multiple different ways to make sure that AI truly understands that this is a refund question and it addresses it accordingly. And so that's something a lot of companies get wrong with AI.
A lot of help desks are saying, oh, yeah, super, super, super easy to configure. It is pretty easy to configure. It's hard to tune really well. And so this is one of the things you want to do is make sure the variations of how to ask the same question trigger the right question, but also get handled based on the context of how they asked it.
And you can accelerate this by doing what we call side by sides with the AI where we're basically you're watching it work and improving it as it goes. So like you're watching what the question is, watching what it thinks the response should be, tweaking that response a little bit and then sending it and basically doing that entire cycle again, just like you would with a new customer service agent. and you are improving it as it goes. And so basically doing side-by-sides with the AI is really effective for building up your knowledge base quickly.
Unfortunately, most AI tools don't do this by default because it's kind of...
You wouldn't know to do that with an AI unless you've trained and managed hundreds of customer service agents over time. And even these software companies haven't done that. And so sometimes you need to build kind of these weird workflows or like separate views of the software, weird technical tools to do that. And that's part of how we've gotten really good results with AI is like we take the tools and the experience we have and the infrastructure we have from training so many actual human customer service agents over the years, over a thousand agents.
And then we apply that to AI. And so either way, what you want to do is make sure you have a really good knowledge base. Lastly, is escalation rate. You want to optimize escalation rate in a similar way as automation rate by, number one, logging the cause for the escalation.
So if a ticket gets escalated up to the human, you want to log the reason for that. The AI needs to log the reason for that. And again, some tools do this, some tools don't. but you can hack around quite a bit of these tools to get what's called a chain of thought output or thinking through, explain your reasoning.
Each tool is slightly different. But what you need to do is get a log of why the AI didn't handle it or why it escalated to human, And then have the human handle the situation, but also update the knowledge base with additional details to deal with that escalation in the future. So again, very, very similar to what you would do with an agent. When a tier one new agent escalates to a tier two agent, a human agent, they don't just say, handle this, right?
They say, I can't do this because of X, Y, Z. The tier two agent then handles it. And typically a team leader will train that tier one agent to not have to escalate that in the future. You want to do the same with AI.
So optimizing and improving AI performance comes down to these specific things that, again, are very similar to humans. And so the key things I want you to understand, and we've got a lot of good questions coming in, Number one, think of AI like a human. Do not think of AI like software. Whether it's in customer service, it's in analytics, it's in accounting, whatever you're doing with AI, don't think of it like a software.
Think of it like a human. It'll help you understand how the AI functions a lot more. Because AI is something that is not... super structured, like typical software has always been of like, if then statements based on a database, like that's not how AI works. AI works based on what's called an objective function.
Like what is the ultimate goal that you want it to do? Then it essentially has the context of everything. That's kind of simplifying it a bit, but it already has all the context and you'll feed it some additional context. You tell it the outcome and then it gets to the outcome is basically what it does.
So that's how a human functions. And so you want to think of it the same way. Second, measure success of AI with customer satisfaction rate of the AI tickets, automation rate and escalation rate. What percentage of the tickets does it completely automate?
What percentage of the tickets it tried to do have to go to a human? And then basically you want to improve those over time. Right. So improve the CSAT score, get it to be eighty percent or higher across human tickets and AI tickets and all tickets together.
The automation rate should be fifty percent or higher and the escalation rate should be below twenty percent. Then what you're going to do is use those metrics to identify areas for improvement and ensure alignment with business goals and then just optimize that over time. Very similar to what you would do on a human team, but doing that on a on the AI tickets. So we're gonna go through some questions, but I'm gonna leave this on the screen so that you guys can check it out.
If you want support in choosing the right AI software, even if you've already tried a few, we can absolutely help. It is such a noisy marketplace. Every help desk is coming out with their own AI tool. Zendesk has it, Gorgias has it.
They're all coming out with variations of tools. There's a lot of third party tools out there. There's Sienna, there's Digital Genius, there's a whole bunch of different ones. The best tool depends on the situation that you're in as a business.
Exactly what type of business you are, exactly what type of e-commerce business you are. Are you on Shopify or Magento or some other stack? Um, what is the help desk that you're on? Uh, is it high ticket, low ticket?
There's a whole bunch of variables that matter to decide which AI software is right. And so we can absolutely help with that. Um, second, if you need a partner that can help you implement it effectively, I hope that, um, you know, what we shared on this call helps you see, like, we understand how to implement this stuff. We've literally built our own AI software.
It's not for the market. We're not going to, we're not getting into the software space, like compete, um, out on the open market as like a self-serve software, but we have built a lot of AI tools ourselves, which again, is very different from other agencies that do this stuff. If you need a partner to implement AI effectively, we can absolutely help. We've used all the tools and we've seen them go through iterations also, so we know which ones are on the right track in terms of what they're doing.
And then if you are kind of at a starting point and you wanna know, you know you wanna start using AI, right? You know you need to, you know you want to, but you're not really sure where to start. Even if you're at the beginning stages, we can help with that too. Even if we don't end up working together, We are happy to have conversations to kind of just point you in the right direction.
And if you're somebody that's on the call that's already pushing AI really, really hard, and maybe you've gotten to this point in the call and said like, I kind of already know most of these things, maybe you've got one or two little nuggets, but like you want to get deep into the weeds, we should absolutely talk. We love nerding out on advanced topics. There's a ton of weird nerd stuff that we haven't touched on here. That's part of why we include that opening segment of like crazy stuff we're seeing in the market.
But if you're already pushing AI hard, we should definitely talk because we we love to kind of work on some of these advanced things But now what I want to do is go into questions. We'll do about ten minutes of questions We've got a couple here in slack. So I'll kind of go through some of these Starting with the first one and what I'll do. I don't know if this will actually help you race L but I am going to paste this into the comments here and I think that'll help you to to highlight it on the screen with the software we're using.
So what other metrics can we look at besides customer satisfaction, automation rate, and escalation rate to see how things are doing? There's a number of different things you could look at, but I do recommend initially just focusing on these ones because that is a holistic enough group of metrics to look at. CSAT is going to tell you if the customers are happy. Automation rate is going to tell you if the AI is handling a big portion of tickets.
And the escalation rate is going to tell you how often humans need to get involved and where the gaps are in your knowledge base. So that's typically going to be the best metrics. Some additional ones you could potentially look at is revenue from customer service tickets and how they vary from for AI driven tickets, for human driven tickets, for AI tickets that gets escalated. You can look at revenue per ticket on those that could potentially help.
You could also look at pre-sale versus post-sale and how CSAT differs for those or how automation rate and escalation rate differs for those. But again, I typically recommend focusing on just those three metrics because that's going to be the foundation for getting AI to work really effectively. The second question that I've got here, what are some ways we can test out new AI features before rolling them out? one of the things I mentioned earlier is doing side-by-sides, right?
With the AI. So watching the AI work and kind of improving it along the way. You can also do mock tickets, right? And every tool does this in slightly different ways, but basically what you're doing is you're taking a real ticket, you're feeding it to the AI and you're seeing what the answer would be, right?
And so that is super, super important to have it have test tickets initially with the AI to see what the response would be like. That will help you to set the knowledge base effectively, to also set the rules for the AI effectively to know which tickets it should handle and which ones should go straight to a human. And then once you've done that, then you can do side-by-sides to say, okay, I'm just going to watch the AI operate. I'm going to let it run, or I'm going to jump in and stop it.
So doing side-by-sides to be able to see how it's operating and manage it in real time. Then you can take it to another level and say, okay, I only want the AI to draft tickets. I'm going to let it do tickets on its own as they come in, but I'm just going to have it draft tickets rather than fully send those responses. And that way you have human eyes on it before the tickets actually get sent.
Then you can get to the point where it actually... uh sends the responses directly uh without you seeing them right um so there's levels to it but again it's it's identical to what you would do with a human agent so test ai the same way you would test a new person on your team you're not going to release them to like all your customers at the same time you're not going to have them send responses to tickets on the first day you might have them do side by sides or do drafts or whatever it is um do it in the same way with ai what I will say is every software does this differently there is a massive company in the space that just released an ai feature um to their help desk And at least when they released it, having conversations with some of their product team and business team, they're very against having it draft tickets.
They do not want it to draft tickets. And the reason why they don't want it to draft tickets is because how do we charge for that? Do we charge for it or do we not charge for it? If it wasn't actually sent but it was drafted, do we get paid for that or not?
The business model is getting in the way of the product. That's fairly common with tech companies, especially if their business model is volume-based with AI. that doesn't change that like you need to vet the tickets first um and so what we've done in that particular case is build like a weird workflow and infrastructure that enables us to basically do side by sides with the agent before it gets sent out and basically drop the tickets um without um having to have that native in their software but That's probably deeper in the weeds than we need to get to. The main thing I want to say here is do side by side to do testing of the AI prior to having it actually send out full responses.
I'm going to take a quick look at these questions to see if there's maybe two more that we're going to handle coming up on time. So I think this is a good one. Let me do this one. So how do we make sure customers feel like they're getting that personal touch even when interacting with AI?
And I'm going to add a layer to this. One of the things that people often get concerned about with AI is, are my customers going to get mad that it's AI? Are they going to feel like they're talking to AI and get frustrated? Are they going to feel like it's a robot response?
Which is a different way to ask the same question. How do you make sure customers feel like they're getting personal responses, even if it's with AI? What we have found... is that when you disclose that it's an AI, the customer satisfaction is worse on the same exact tickets, the same scenarios, than if you don't disclose that it's an AI. So if you don't disclose that it's an AI, the performance, the customer satisfaction, the customer's feeling of was this good, uh is better if you don't disclose that it's an ai and I think the reason for that is that customers are preconditioned to think the ai results are going to be poor they're preconditioned to say oh my god this is just a chatbot oh my gosh this is just uh this is just an automated response or self-service response or something um there has been chatbots and things like that in the past and they've been typically pretty bad And now there's AI, which initially was like a glorified chatbot.
And now AI is insanely good if you know how to tune it. But customers still feel like if they see that it's an AI, they assume it's going to be crappy, right? And so what we have found is the right recipe is to not disclose that it's an AI, have AI take the initial tickets essentially, depending on which tickets you're having it do, but don't disclose that it's an AI and then get the escalation logic the decision process for the AI to escalate to a human, get that tuned well so that before the human starts feeling friction, the AI says, this is going to need to go to a human agent. And then it escalates to a human.
It looks like it just transferred to another human agent. Let me check with Sarah. I think she's going to need to dig into this. Then it goes to Sarah.
Sarah is actually a human, but the thing that transferred to Sarah is not. It's the AI. And then Sarah solves it, and that's it. That makes customers feel like they're getting really good service.
It makes customers feel like they're getting that personal touch. It is something programmatically that you can do fairly simply, like having escalation logic built in. but you haven't disclosed that it's an AI. And so that is what we have found works best because if you disclose that it's an AI immediately, many, many customers at all, but many customers will assume it's going to be crappy and sometimes cause the experience to be poor.
by saying talk to a human or something right away, just because they think it's going to be bad. So what I'm trying to say is to get that right personal touch, even if they're interacting with an AI, I think the tactical thing I'm telling you is test not disclosing that it's an AI and make sure that you have that escalation rate really, really effective. With that said, there are some legal and regulatory things you need to be aware of. Different states seem to handle it differently.
I'm sure different countries handle it differently as well. So you do need to pay attention to the regulatory environment. Unfortunately, the regulatory environment hasn't really caught up with technology as they normally don't. So you need to make sure that you're meeting the regulatory guidelines and probably doing it differently state by state.
Again, that's a little complicated to do, but at a strategic level, what we have found is not disclosing makes it perform better for the customer. And I think that's going to be the last question. Let me do one more, actually, the training one, and then we will wrap up on this. If there's any other big questions that come up, put it in the comments.
Ray, Raysell, if you can put that in Slack real quick, but this will be the last one otherwise. What is the best way to train our AI tools to handle more complex customer service issues? The answer to this is to treat the AI just like a human. That's the answer to most questions.
When the AI responds... you need to do QA, quality assurance on those tickets. You need to do it on human ones too, but you need to do QA on those actual tickets and say, okay, did it respond correctly according to the customer's questions? Did it use the knowledge base that we have? Did it phrase the question correctly?
Did it use empathy? All these different things, right? You need to QA those tickets and then improve the knowledge base and improve the AI configuration based on those tickets, just like you do with a human. And so by doing that, you will start to improve the knowledge base so that it can handle more complex scenarios.
Not only will you be able to add scenarios that it will handle, but you also be able to tune the actual scenarios that the AI handles to handle the granular differences between them. So remember at the beginning, I talked about VIP customers and how you might want to treat those refunds differently, those refund requests. You could do that in the knowledge base over time once you start to see those patterns of how the AI is handling it. So the way to train AI to handle more complex situations is just like a human, review all of the AI tickets and then improve the knowledge base based on those.
This is an example of something we've done and all companies should really be doing with AI. So we built a QA tool. It's been quite a while. It's been over a year, I think a year and a half.
But basically a QA tool that analyzes all the chats and all the tickets of not only humans, but also of AI, analyzes all of them and conducts the QA process automatically. So we're able to QA a massive portion of tickets automatically. using AI by training the AI to do the QA process, just like our QA specialists have done. And so that gives us the ability to not only process and do tickets with AI, like we're talking about today, but also to QA those tickets using an AI driven process.
So it's been really, really effective in terms of improving the AI to handle a lot of customer service. So that's going to be what we'll dig into for today. Again, if you want some support from us, visit HelpFlow.com. We can help you on getting the right AI software in place, configuring AI, using AI effective.
We have run customer service for hundreds and hundreds of brands. Like I mentioned at the beginning, we've been doing it for ten years. So that's a long time in Internet time. And we have a big team.
And also our team is very, very powerful. technical that's something I'm most proud of with our team is we went we have we've always been technical from the beginning but there's just something inside us that got excited with ai even though ai is changing customer service so much and it's absolutely disrupting like what our business used to be but we're just excited by technology we've always been excited by technology and that puts us in a unique position to be able to absolutely maximize the use of ai in a customer service space I truly believe that the companies that are going to be best positioned to maximize ai and automate as much of customer service as possible are customer service agencies that know their tech stuff really really well and that is us and we put ourselves in this position to really help brands and companies like you maximize AI.
So if you need help or if you just want to dig into AI and how to best use it in your business or just customer service in general, visit Helpflow.com. We can set up a call with my team. And again, no pressure. If we don't work together, it's not a big deal.
You'll get a ton of value by going through the process. And we just like connecting with other people in the space and frankly, just learning more and more about how how to apply AI effectively in different businesses. So visit helpflow.com, schedule a call.
I hope you got a lot of value out of today's session and make sure that you're on the email list for future sessions. We're going to be doing a lot more of these webinars so that we can teach you how to use AI effectively in your business, whether it's in customer service or other situations. But for now, I hope this has been helpful and we'll talk to you soon. Thank you so much.
Jon Tucker
- Website: https://www.ecommercetownhall.com/
- Email: jtucker@helpflowchat.com
- Facebook Page: https://www.facebook.com/JonTuckerUSA
- Twitter/X: https://x.com/JonTuckerUSA
- Linkedin: https://www.linkedin.com/in/jontuckerusa/
Ecommerce AI Mastermind
How The AI
Mastermind Works
This will be a laid-back, no-pitch session where you can connect, learn, and discover actionable AI tactics. Expect a lively discussion filled with actionable insights that'll give you those "aha moments." You’ll walk away with innovative AI strategies you can directly apply to your business, ready to spark real change.
Next Ecommerce AI Mastermind Session
11
22
38
32
Meet Your Host
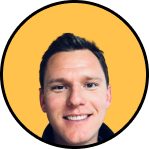
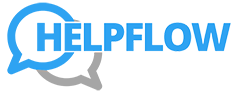
Jon Tucker is CEO of HelpFlow - and a huge nerd when it comes using AI effectively. Jon has been leading the charge at HelpFlow in guiding our client brand partners to leverage AI over the past 2- 3 years. In addition to client use cases, Jon has spearheaded numerous ways that HelpFlow applies AI behind the scenes in our operations - so he knows how to apply AI in a wide range of business scenarios.
When it comes to the AI Mastermind, Jon is the perfect moderator to maximize insights and impact. Not only is he deep in the AI rabbit hole, but he's got years of experience being a member and leader of various business groups. With this nerdy knowledge of everything AI + ability to drive a group discussion, these masterminds are sure to be valuable for everyone that attends.
Ready to dive in? Register today!
Be A Guest on our Mastermind or Podcast
Contact us now if you are:
- An Ecommerce Brand with an inspiring story and best practices to share.
- Or a Tech Company who wants to showcase their technology as a useful ecommerce solution.